May 2, 2025
Arelis AI
Building an Effective AI Governance Framework for the EU AI Act
A step-by-step guide to balancing innovation with risk management
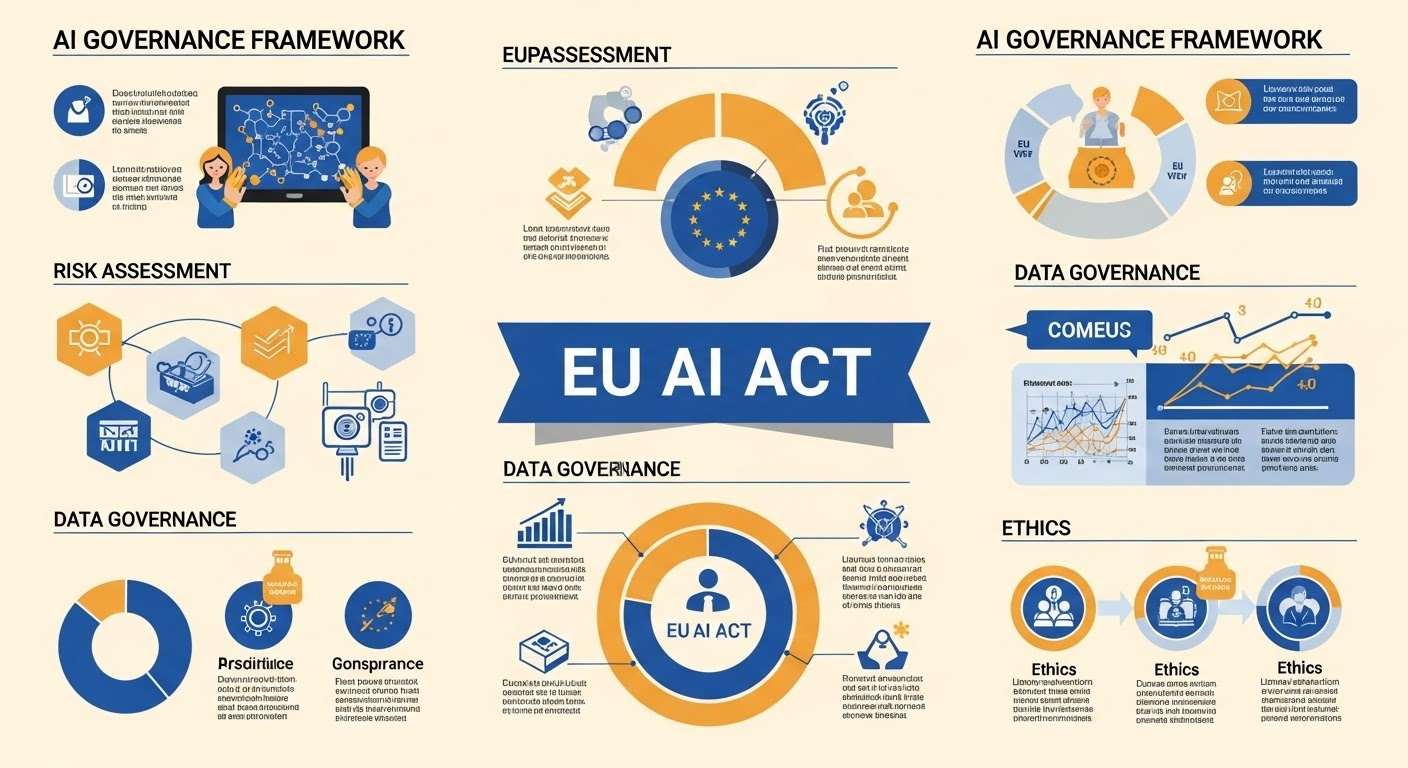
Read time: 15 minutes
Summary
The EU AI Act, effective August 2024, is the world's first comprehensive AI regulation with strict compliance deadlines. This guide provides a practical framework for organizations to navigate the Act's requirements, from initial risk classification through full implementation. Key elements include understanding the four-tier risk classification system, implementing required governance structures, and following a phased roadmap with critical milestones in 2025-2027. The article offers actionable steps for building compliant AI systems while maintaining innovation velocity.
1 | Why the EU AI Act matters now
Over the past five years, artificial intelligence has moved from research prototypes to the nervous system of European business and government. Generative models draft policies and marketing copy; computer-vision engines police factory floors; recommendation algorithms shape political debate. In early 2024 EU lawmakers responded to that reality with the world's first horizontal AI law, the Artificial Intelligence Act (AI Act)—legislation designed to embed safety, fundamental-rights protection and market confidence into every stage of the AI life-cycle.
The law cleared its final political hurdles in record time: Parliament adopted the deal struck in trilogue on 13 March 2024, and the Council gave its unanimous "green light" on 21 May 2024. Publication in the EU's Official Journal followed on 12 July 2024, triggering a fixed countdown: the Act entered into force on 2 August 2024, six months after signature.
That start-date matters because the Act's obligations roll out on a tight, staggered schedule. Banned practices—such as social scoring or manipulative subliminal techniques—must disappear from EU markets by 2 February 2025. General-purpose AI (GPAI) transparency duties follow at the one-year mark, and the heavy-weight high-risk obligations (quality-management systems, data-and-bias controls, conformity assessment) bite 24 months after entry into force, on 2 August 2026. Some sector-specific high-risk systems get an extra year, pushing their deadline to 2027.
For organisations, the message is clear: compliance is no longer an optional audit exercise deferred to year-end. The AI Act rewires product-development pipelines, vendor contracts, incident-response playbooks and board-level risk reporting. Companies that mobilise early—treating governance as an enabler of trusted AI products rather than a legal cost—will shape emerging market norms and secure a first-mover advantage with regulators, investors and customers alike.
2 | What the AI Act actually demands
A wide territorial and functional reach The regulation applies to anyone who places, puts into service or uses an AI system in the EU, even if the provider is established outside Europe. It also captures situations where the system's output is used in the Union, giving the Act genuine extraterritorial bite. The main regulated roles are providers (developers or vendors), deployers (users), importers, distributors, product manufacturers that integrate AI, and authorised representatives.
Every AI application must be classified into one of four tiers:
- •
Unacceptable risk – practices banned outright because they contravene fundamental rights or democratic values (e.g. social scoring, untargeted scraping of facial images, manipulative subliminal techniques).
- •
High risk – systems that can affect life, health, safety or fundamental rights (credit scoring, recruitment, essential public services, critical infrastructure, medical devices, etc.).
- •
Limited risk (transparency risk) – systems that interact directly with people or generate synthetic content (chatbots, virtual assistants, deep-fakes, generative text meant for public information).
- •
Minimal or no risk – all other uses, encouraged through voluntary codes of conduct.
Core duties for high-risk systems
Once a model is tagged "high risk", providers must operate a documented Quality Management System (QMS) under Article 17; maintain detailed technical documentation; implement robust data-governance and bias-mitigation controls; carry out pre-market conformity assessments (internal or by a notified body, depending on the use case); affix CE marking; and create a post-market monitoring plan. Deployers must use the system in accordance with the provider's instructions, carry out context-specific risk assessments, and log events for 6–10 years.
Serious-incident reporting and continuous vigilance
If a high-risk AI system causes—or is likely to cause—a breach of fundamental rights, providers (and in some cases deployers) must notify the competent authority within 15 calendar days of becoming aware of the incident, then co-operate on corrective actions. Article 72 also obliges continuous performance monitoring to catch model drift, degradation or harmful emergent behaviours.
Tailored transparency for limited-risk uses
Systems that merely interact with people or generate/manipulate content must tell users they are dealing with AI, and label synthetic media clearly enough that an average person understands it is machine-generated. These duties sit in Article 52 and apply from the moment the system is put on the market—long before the heavier high-risk rules kick in.
Obligations for general-purpose AI models (GPAI)
Foundation-model providers must publish technical documentation, a plain-language model card describing capabilities and limitations, and a summary of the copyrighted data used for training. Highly-capable GPAI models that pose systemic risk must go further, performing model-evals, incident simulation and adversarial red-teaming, then reporting the results to the new EU AI Office. These transparency obligations start one year after entry into force—that is, August 2025.
Enforcement with teeth
Non-compliance can trigger administrative fines of up to €35 million or 7 % of global annual turnover for prohibited practices, €15 million/3 % for breaches of high-risk obligations, and €7.5 million/1 % for supplying false information. Regulators may also order systems off the market and publish public-naming sanctions.
Taken together, these provisions replace today's ad-hoc "AI policy" checklists with a legally binding operating system for innovation. Classify accurately, build the required controls into everyday engineering workflows, and document everything from design choices to deployment logs—you will have met the letter of the law and created a sturdier platform for trusted AI products.
3 | A step-by-step governance blueprint — from the boardroom to the build-pipeline
Building EU-grade AI governance is less about stapling a new policy onto the end of software delivery and more about rewiring who decides what at every stage of the model life-cycle. Below is a sequenced playbook that has proved workable for both multinationals and resource-constrained scale-ups.
3.1 Secure an executive mandate and ethical north-star
Start with a formal board resolution that treats AI as both a strategic asset and a regulated risk class. Pair that mandate with a concise set of ethics-by-design principles—lawfulness, fairness, transparency, robustness, privacy and ecological sustainability—and hard-map each principle to a board-level KPI (e.g. percentage of models with completed bias impact assessments). This "tone at the top" unlocks budget and signals to teams that compliance is inseparable from product success.
3.2 Stand-up a cross-functional governance architecture
Create a permanent AI Governance Committee that brings Risk, Legal, Data Science, IT, Product and HR to the same table. Give each role non-overlapping accountability: business owners define intended purpose and user population; model owners manage day-to-day performance; a single AI Governance Lead orchestrates artefacts and makes go/no-go calls; Internal Audit provides independent challenge. Medium-sized enterprises that cannot staff a full committee can outsource second-line functions to an external conformity-assessment body on retainer.
3.3 Run a bottom-up AI inventory and risk classification
Most organisations underestimate how many scripts, SaaS features or vendor APIs qualify as "AI". Conduct a comprehensive census—shadow IT included—capturing intended purpose, training data lineage and potential rights impact. Classify each system against the Act's four-tier taxonomy, flagging "uncertain" cases for provisional high-risk treatment until legal counsel confirms otherwise. Early classification avoids late-cycle redesign and supports capital-allocation decisions.
3.4 Design a Quality Management System that meets Article 17
For every system that lands in the high-risk bucket, Article 17 demands a written Quality Management System (QMS) that spans design controls, data governance, human oversight, record-keeping and incident handling. The fastest way to satisfy the article is to adopt ISO/IEC 42001:2023, the new AI-management-system standard, as the scaffold for policy and procedure libraries. The annexes of that standard supply template process maps, audit check-lists and metrics, reducing drafting time by months.
3.5 Engineer data-governance and bias controls up-front
A QMS without data governance is window-dressing. Borrow controls from ISO/IEC 23894:2023, which sets out concrete risk-assessment techniques—differential privacy, federated learning and data-minimisation patterns—well aligned with the Act's expectations on training-data quality and representativeness. SMEs can embed these checks into open-source MLOps pipelines such as Great Expectations or Evidently so that evidence is generated automatically during model training.
3.6 Make transparency and human-centric design non-negotiable
At each major stage gate—requirements, architecture, testing—developers should attach a short "model card" that describes the system's purpose, capabilities, limitations and evaluation data sets in plain language. For high-risk use cases, augment these cards with a human-oversight plan that spells out when a human can interrupt or override the model. Drafting these artefacts while the code is still in the pull request avoids post-hoc guesswork and meets the Act's explicit documentation duties.
3.7 Industrialise testing, validation and documentation
Replace ad-hoc Jupyter experiments with a standard validation protocol covering functional accuracy, robustness to adversarial inputs, fairness metrics and resilience to concept drift. Bake the protocol into the CI/CD pipeline so that promotion to production is blocked until all benchmarks and autogenerated documentation are uploaded to the QMS repository. Store versioned model artefacts and validation logs for the 6–10 year retention period the Act prescribes.
3.8 Operationalise post-market monitoring and incident response
Article 72 turns real-world monitoring from a "nice to have" into a statutory duty. Implement telemetry pipelines that stream key performance indicators—accuracy, false-positive/negative rates, fairness drift—to a dashboard owned by the Governance Committee. Define statistical-process-control thresholds that trigger automated retraining or a manual "kill switch." If a serious incident occurs, the provider has just 15 calendar days to notify the competent authority, so pre-approved templates and 24/7 on-call procedures are essential.
3.9 Close the loop with audit and regulator dialogue
Plan an internal audit at least annually, prioritising models with higher societal impact. Where a notified body is required, schedule its conformity assessment early to avoid a bottleneck with other market actors racing toward the same 2026 deadline. Keep a briefing pack ready for the new EU AI Office—now empowered to run EU-wide sweeps and receive complaints under Article 85—so the organisation can demonstrate continuous control even under tight notice.
3.10 Embed continuous improvement and a culture of responsible AI
Governance that relies on static playbooks will fail the moment a model drifts or a new large-language-model API appears. Run quarterly "red-team" exercises to probe for emergent risks; publish the lessons learnt internally; and refresh policies in an agile increment rather than annual rewrites. Track operational metrics—mean time to detect drift, bias-incident frequency, documentation cycle time—to show the board how maturity is improving quarter over quarter.
4 | Enablers and accelerators — shortening the road to trustworthy AI
A strong governance blueprint still needs fuel in the tank. The EU AI Act itself names several "facilitating measures," and early adopters have already learned which catalysts move the maturity needle fastest. Below are six practical accelerators that convert policy intent into day-to-day engineering reality.
4.1 Industrial-grade tooling: registries, doc-bots and compliance checkers
Paper templates can't keep up with CI/CD velocity. Modern teams are plugging cloud-native "AI registries" into their pipelines so every model automatically receives a unique ID, version, risk label and evidence bundle.
4.2 Regulatory sandboxes: a "safe room" for bold experiments
Article 57 compels every Member State to launch at least one AI regulatory sandbox by August 2026. Inside these supervised playgrounds providers can test novel or high-risk systems with real users while receiving live feedback from data-protection and market-surveillance authorities. Participation isn't just academic: the documentation generated in a sandbox can later substitute for parts of the formal conformity-assessment dossier, a major time-saver for SMEs.
4.3 External assurance and fast-track certification
Most companies lack the bandwidth to audit their own AI stack objectively. Early movers are therefore pairing an internal ISO 42001-style QMS with third-party assurance from certification bodies and notified bodies already gearing up for AI assignments. Interest is surging: KPMG and LRQA report wait-lists for "early-adopter packs" that bundle readiness assessments with formal audits once the market is fully designated. The Commission will soon publish a live list of designated notified bodies, allowing firms to book slots before the 2026 rush.
4.4 Offensive security and red-teaming tools
The EU AI Act's systemic-risk and post-market-monitoring requirements assume that providers can proactively identify and address failure modes—before users encounter them. Arelis AI embeds adversarial evaluation capabilities directly into its enterprise-grade platform, enabling continuous red teaming against integrated LLMs and AI agents. This includes simulated prompt injections, jailbreaks, and data poisoning scenarios aligned with compliance and risk classification under the EU AI Act. These built-in mechanisms allow Arelis to generate the red-team and audit reports that the AI Office will expect from high-impact GPAI systems, turning compliance into a repeatable engineering discipline rather than a one-off burden.
4.5 Deep stakeholder engagement
Technical correctness is not enough; the Act's recitals stress fundamental-rights impact. Forward-looking organisations convene civil-society groups, accessibility advocates and affected user cohorts at design-stage workshops, then publish the feedback loop alongside model cards. This practice costs little, pre-empts reputational crises and demonstrates the "participatory" ethos EU regulators now prize.
4.6 A disciplined change-management playbook
Even the best controls die in desk drawers without behavioural change. Successful programmes treat governance rollout like a product launch: executive town-halls to frame the narrative, role-based micro-learning modules delivered in the IDE, and quarterly "AI Day" hackathons where teams practice sandboxing, bias testing and incident drills. Tracking metrics such as documentation cycle-time or mean time to mitigate drift keeps attention on outcomes rather than binder thickness.
Put together, these accelerators collapse lead-times, build organisational muscle and turn compliance artefacts into living assets. Deploy them in parallel with the governance steps outlined in Section 3, and you'll arrive at 2026 not merely ready for the AI Act—but ahead of the competition in shipping trustworthy, high-performing AI products.
5 | Implementation roadmap — from "kick-off" to Day-2 compliance
The EU AI Act imposes hard dates that anchor any corporate workplan. Entry into force on 1 August 2024 set four statutory checkpoints:
- •February 2025 ( + 6 months ) – prohibited practices must cease.
- •August 2025 ( + 12 months ) – GPAI transparency and AI-literacy duties apply.
- •August 2026 ( + 24 months ) – core high-risk obligations bite for Annex III use-cases.
- •August 2027 ( + 36 months ) – extended deadline for safety-component AI and certain sectoral systems.
Below is a phased roadmap that back-plans from those dates while allowing for the realities of budget cycles and vendor lead-times. Think of each phase as a gate: do not progress until all minimum deliverables are complete.
Phase 0 | Mobilise (Aug–Oct 2024)
- •Secure a board-level mandate, appoint an AI Governance Lead, and define internal checkpoints.
- •Publishing a one-page charter that aligns with legal milestones ensures stakeholders remain engaged.
Arelis can accelerate this phase with off-the-shelf governance dashboards and templated charter tools, helping teams align on scope early.
Phase 1 | Discover & classify (Nov 2024 – Jan 2025)
- •Run a full AI census, including shadow IT.
- •Tag risks, label systems provisionally, and compare to legal baselines.
Arelis' AI system registry and classification toolkit streamlines this phase—offering traceability and audit trails critical for mapping Article 6–9 obligations.
Phase 2 | Design (Feb – Apr 2025)
- •Draft QMS blueprints aligned to ISO standards.
- •Build documentation templates and choose tooling for privacy and explainability.
The platform's pre-configured model cards, risk templates, and bias assessment forms in Arelis' governance module save time and standardize across departments.
Phase 3 | Build & pilot (May 2025 – Mar 2026)
- •Connect registries and audit logs to CI/CD.
- •Pilot a high-risk use-case, engage a sandbox, and run adversarial evaluations.
Arelis' integrated logging, sandbox capabilities, and red-team support features allow organizations to capture regulator-grade evidence by default, not exception.
Phase 4 | Conformity & rollout (Apr – Jul 2026)
- •Conduct internal assessments and prepare for notified-body audits.
- •Deploy CE-marked systems with production-grade controls.
The compliance dashboard in Arelis enables real-time conformity tracking and simplifies documentation ahead of audits.
Phase 5 | Sustain & extend (Aug 2026 – Aug 2027)
- •Operationalize drift detection and KPI alerts.
- •Expand workflows to safety-critical AI.
Arelis supports ongoing risk monitoring with performance dashboards and serious incident protocols tailored for Annex III systems.
Phase 6 | Culture & optimisation (ongoing)
- •Role-based training, policy iteration, and executive reporting.
With its built-in training module and governance analytics, Arelis makes it easy to embed continuous learning and compliance metrics into daily workflows.
Key takeaway Back-planning from statutory deadlines converts the AI Act from an abstract legal risk into a manageable delivery programme. Organizations that anchor governance early—especially with platforms like Arelis AI—can turn compliance into a strategic enabler of scalable, trusted AI.
Related Articles
Stay Updated
Get the latest insights on AI governance and compliance delivered to your inbox.
Subscribe to Newsletter